2024/5/20
DeepEyeVision Inc.
Citadel AI Inc.
Citadel AI Inc. (HQ: Shibuya, Tokyo; CEO: Hironori “Rick” Kobayashi; hereinafter referred to as “Citadel AI”), a leader in trustworthy AI technology, and DeepEyeVision Inc., a med-tech startup developing AI-based computer-aided diagnosis systems for ophthalmic images, originating from Jichi Medical University (HQ: Shimotsuke, Tochigi; CEO: Hidenori Takahashi; hereinafter referred to as “DeepEyeVision”), conducted joint research to further advance the performance and safety of AI in ophthalmology. The results were presented at the 128th Annual Meeting of the Japanese Ophthalmological Society held from April 18 to 21, 2024.
Challenges in AI-Based Computer-Aided Diagnosis
Medical AI has the potential to provide innovative healthcare solutions across diagnostic support, optimization of treatment plans, and patient monitoring. In particular, AI used in computer-aided diagnosis, which assists in interpreting medical images, can alleviate the geographic and resource constraints of radiologists, achieving a more equitable supply of image diagnostics.
However, AI-specific engineering challenges still pose an obstacle to the adoption of AI in clinical settings. For example, insufficient data quality used in training models can lead to incorrect results in real clinical settings. Moreover, if there is bias in the training data, AI models trained on such data are known to produce biased predictions.
By tackling these engineering challenges when developing models for computer-aided diagnosis systems, we can deliver more reliable AI to medical professionals and patients, and advance the adoption of medical AI systems.
Importance of Evaluating AI Robustness
DeepEyeVision focused on the impact of image perturbations (such as noise associated with the imaging environment of retinal images captured by retinal cameras and image processing pipelines) on AI predictions in its joint research with Citadel AI. For example, the left retinal image below shows a condition called macular degeneration. The AI was able to correctly classify the original image, but when the image was slightly blurred, the AI failed to make a correct prediction and determined that there was no disease. Such subtle changes in images can occur in clinical settings due to changes in the internal configuration of retinal cameras or changes in the external imaging environment.

This joint research examines the robustness of a multi-label classification AI model for predicting diseases in retinal images, i.e. how resilient the AI is to perturbations in its input. Based on these results, DeepEyeVision also refined its AI training process and evaluated the outcome of these training modifications.
Joint Research on AI Robustness in Ophthalmology
In this joint research, DeepEyeVision first trained a multi-label classification model using approximately 400,000 normal-angle color retinal images of 50 diseases collected through appropriate medical ethics procedures at Jichi Medical University. Then, they evaluated the AI model’s robustness on synthetic perturbations in retinal images using the image robustness test suite in Citadel Lens.
The top left image is the original retinal image, followed by images with increased contrast, decreased contrast, optical distortion, zoom blur, increased brightness, decreased brightness, and zoomed-in retinal area.
Next, we inputted these perturbed retinal images into the AI model and compared the predictions with those of the original retinal images. In this experiment, we used a metric called the Robustness Score, which represents the degree of change in predictions across the entire dataset before and after adding perturbations. A higher Robustness Score indicates that the model has strong robustness against those perturbations.
For this joint research, we used Citadel Lens to evaluate, visualize, and further improve the quality of this AI model.
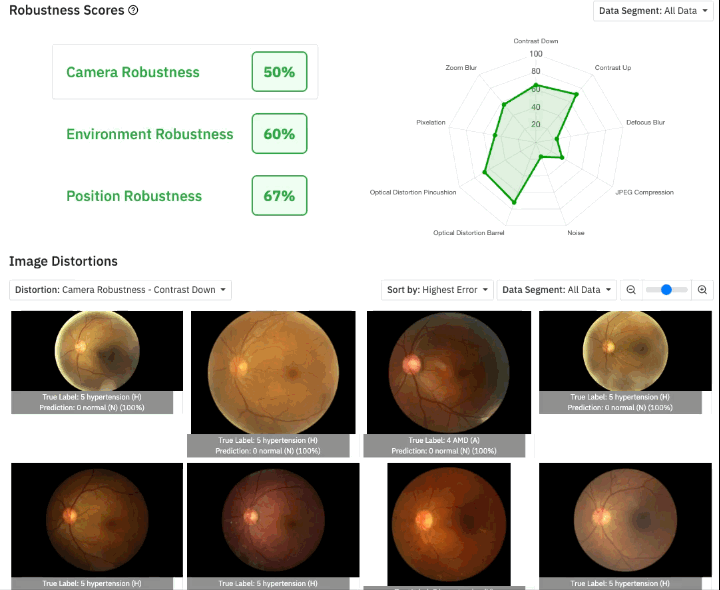
The evaluation results from Citadel Lens revealed that the original AI model developed by DeepEyeVision had particularly low scores in the “Brightness Down” category, meaning that its predictions were significantly affected by low brightness. Therefore, we proceeded to retrain the model on additional data with varying levels of brightness to simulate environmental perturbations.
The results are summarized in the table below, comparing the robustness of the original model with the model trained on data with a wider range of brightness levels. The second, retrained model exhibited higher robustness scores to brightness perturbations, meaning that it maintained better performance under changes in brightness.
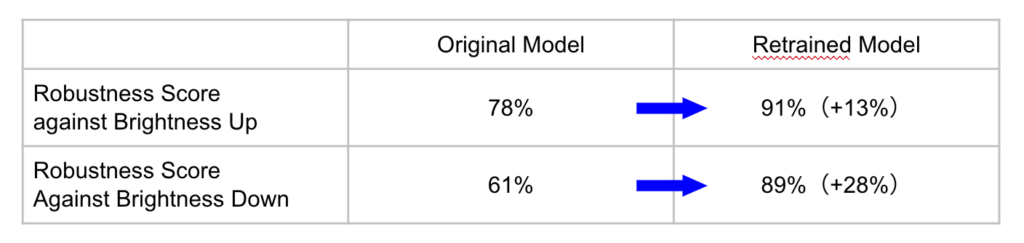
Furthermore, as shown in the chart below, the retrained model also improved robustness across a wide variety of perturbations beyond just brightness. In the future, DeepEyeVision plans to expand the set of perturbations during training and further refine model performance.
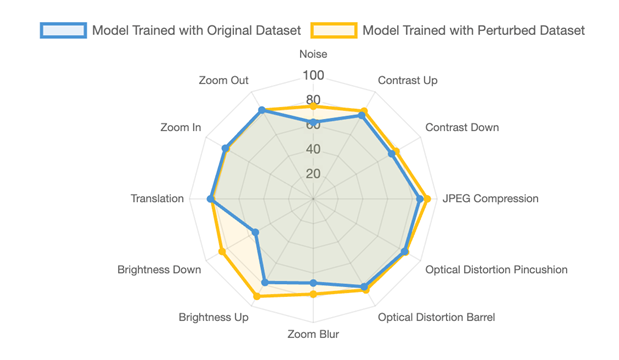
For more details, please find the details of the research here: Improving AI Reliability in Ophthalmology.pdf
Comments from Yusuke Kondo, CTO of DeepEyeVision
The AI-powered medical devices developed by DeepEyeVision have already obtained regulatory approval as medical devices for multiple products and are being used in actual clinical settings. We frequently receive requests from ophthalmologists who use AI in their daily practice, praising its convenience while also expressing a desire for enhanced robustness and explainability of AI. Citadel AI, led by CEO Hironori Kobayashi, who, like myself, graduated from the Department of Electrical Engineering at the University of Tokyo, has world-class technical capabilities in AI reliability assessment technology. In fact, by utilizing Citadel Lens, we were able to achieve improvements in robustness and explainability as demonstrated in this presentation. To disseminate high-quality ophthalmic AI worldwide, we will continue to work closely with partner companies and organizations.
Comments from Kenny Song, CTO of Citadel AI
Citadel AI’s products encompass various functionalities necessary for enhancing the safety and reliability of medical image AI. By utilizing Citadel Lens, DeepEyeVision was able to conduct comprehensive and automated testing of AI, including robustness tests used in this study, as well as automatic detection of data bias, estimation of label errors, fairness testing, and visualization of explainability, among others. Building on the results of this joint research, DeepEyeVision and Citadel AI plan to further collaborate for the long-term development of AI in the medical field. Additionally, both companies are members of the AI Medical Device Council, where they strive to share industry best practices and advance the implementation of AI medical devices. We aim to provide a positive impact to society and the industry as a whole.
About DeepEyeVision
DeepEyeVision Inc. is a venture-backed startup established by Dr. Hidenori Takahashi (Ph.D.), an associate professor at Jichi Medical University, with the aim of delivering medical AI technology from academia to society. Utilizing AI-based image diagnostic technology, the company conducts research and development with the mission of advancing various medical fields beyond ophthalmology.
CEO | Hidenori Takahashi |
Headquarters | Jichi Medical University Ophthalmology Lecture Hall, 3311-1 Yakushiji, Shimotsuke City, Tochigi Prefecture 329-0498 |
Establishment | May 10, 2016 |
Second-class medical device manufacturing and sales | License number 09B2X10014 |
Medical device manufacturing | Registration number 09BZ200083 |
Company URL | https://deepeyevision.com/ |
Inquiries | pr@deepeyevision.com |
About Citadel AI
CiCitadel AI provides software products that test and monitor the quality of AI systems. Our technology helps organizations minimize AI reliability risks and maximize AI performance from research to deployment. Citadel AI’s technology is built from our team’s first-hand experience deploying high-risk AI systems at world-leading companies such as Google Brain, Waymo, Toyota, and more.
Representative Director | Hironori Kobayashi |
Headquarters | Shibuya-ku, Tokyo |
Establishment | December 10, 2020 |
Company URL | https://www.citadel.co.jp |
https://twitter.com/CitadelAI | |
Contact us | info@citadel.co.jp |